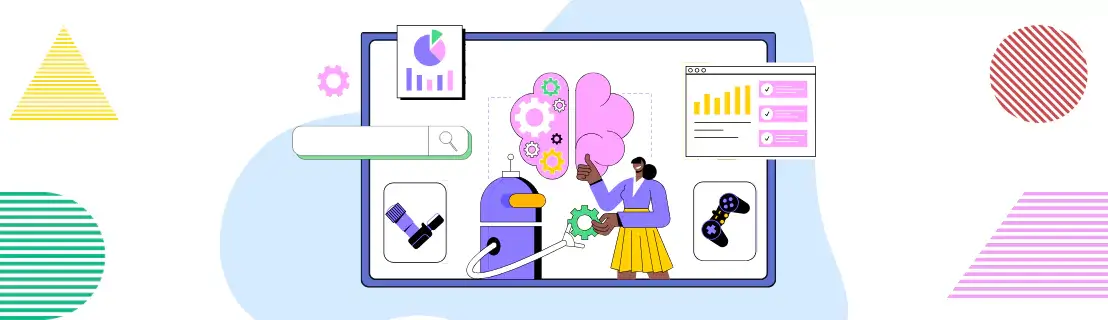
AI suggestions are everywhere in eCommerce. They help improve customer experience and increase sales by presenting the right products at the right time. A study by Accenture revealed that 84% of C-level executives from around the world view AI as the key to meeting business goals.
From personalized product recommendations to dynamic search results, AI suggestions are delivering tailored experiences to keep your customers engaged. In this post, we will learn more about AI suggestions, how they work, and how you can utilize them to enhance your eCommerce store.
AI Suggestions in ECommerce - What Does it Mean?
AI suggestions in eCommerce are data-driven recommendations generated by an intelligent AI engine to enhance shopping experiences. To do so, AI employs various algorithms like product search algorithms, ML algorithms, and natural language processing (NLP) to identify patterns in customer behavior and automatically recommend products that match their preferences.
Besides customer behavior, AI can assess and evaluate customer data like demographics, preferences, wish lists, browsing history, and so on to customize search results and promotional offers to boost product discovery.
That being said, let us explore the benefits of integrating AI suggestions into your online store.
Better engagement - AI suggestions deliver highly relevant product recommendations to customers. This level of personalization captures their attention and encourages them to explore more products. It increases the amount of time they spend on your website keeping them engaged and deepening their shopping journey.
Higher conversions - AI suggestions eliminate the friction from the buying process by showcasing relevant products to customers at the right time. With the help of tailored suggestions, they are guided toward the products they are more likely to purchase, thereby increasing conversion rates. Studies have revealed that AI recommendations can contribute to a 15-20% increase in conversion rates if rightly implemented.
Enhanced user experience - AI suggestions eliminate the guesswork and reduce decision fatigue for customers by simplifying the shopping journey. Thanks to intuitive product recommendations, customers do not have to navigate through hundreds of product listings to find what they want. It anticipates the customers' needs and builds a smooth shopping journey that not only delivers value but also saves time.
Increased sales - AI suggestions ensure that you do not miss any sales opportunities. It does so by strategically suggesting premium products or complementary items to customers, encouraging them to add more items to their carts. This results in larger cart values and overall increased sales.
How Do AI Suggestions Work?
An AI suggestions system is usually associated with sophisticated algorithms that use big data to recommend products to customers. Big data is nothing but large data sets that include various details -
- Customer interactions like browsing history, past purchases, clicks, and so on.
- Product information like product titles, descriptions, features, and so on.
- Contextual data like customer location, device, time, and more.
These recommendation systems are trained to understand and interpret customer preferences and past decisions with the help of the big data that is gathered from their interactions with your store. To do so, the recommendation systems follow different models or approaches for suggesting products.
Types of Recommendation Models
Most commonly used recommendation models fall into these broad categories -
1. Content-Based Filtering
This recommendation model suggests products that are similar to those the customers have interacted with in the past. It relies heavily on product metadata. That is, it analyzes different characteristics of the products like brand name, color, material, price, and so on to compare them to the items customers have shown interest in.
For example, if a customer buys a blue dress, the recommendation system will identify other blue dresses or similar fashion items and include them in the suggestions.
This type of recommendation model is ideal for offering personalized suggestions to customers based on their individual preferences.
2. Collaborative Filtering
This recommendation model focuses solely on customer behavior rather than product features or product metadata to suggest relevant products. It analyzes behavior patterns across many customers to identify common behavioral patterns that could be unique to customers having similar preferences. It assumes that such customers would prefer receiving similar product suggestions.
Collaborative filtering can be further categorized into two types -
User-based collaborative filtering - To recommend products based on what similar customers liked. For example, If you have purchased a blue dress in the past, the recommendation engine would find other customers who bought the same blue dress only to realize that they purchased a pair of matching blue heels. It assumes that, since you liked the same dress as those customers, you would be interested in blue heels and thus, suggests the same to you.
Item-based collaborative filtering - To recommend products that are similar to what customers purchased in the past. For example, if you purchased a blue dress, the recommendation engine will look for other items that are commonly purchased along with the blue dress. Let's say the customers who purchased blue dresses also purchased gold heels and a handbag. The system will then suggest both items to you.
The collaborative filtering recommendation approach generates diverse product recommendations. Hence, it is ideal for introducing customers to new products that they might have not considered.
3. Hybrid Filtering
The hybrid filtering recommendation approach utilizes both content-based and collaborative filtering approaches to create more accurate product recommendations. It takes into consideration product metadata as well as customer behavior to deliver product suggestions ensuring better relevancy.
Continuing with our example, if you have already purchased a blue dress, the hybrid recommendation engine will suggest a pair of matching blue heels (content-based suggestion) and a gold handbag (collaborative filtering suggestion) that other customers have bought alongside the dress.
This type of recommendation approach offers the benefits of both methods - content-based filtering and collaborative filtering to offer more accurate and diverse product recommendations.
Understanding the AI Suggestions Process
AI suggestions follow a structured process to deliver personalized experiences. Let us take a look at this process in detail.
Data gathering - The AI suggestions engine collects data from various sources - customer behavior, product details, and more. Remember data plays an important role in AI suggestions. The more data the system collects, the better tailored the suggestions will be.
Data preprocessing - The next step is to clean the raw data that is collected to remove any inconsistencies or errors. This process converts and structures your data into usable formats for machine learning algorithms to show their magic.
Model development and training - AI systems use various models, such as content-based filtering, collaborative filtering, or hybrid filtering. During this step, the AI suggestions engine is trained to identify patterns within the data and make predictions on which products a customer is more likely to purchase based on their past actions. The model is now ready for action.
Real-time Analysis and Suggestions - As a customer interacts with your store, the AI model processes product data and customer behavior in real time to generate relevant recommendations.
Continuous learning and optimization - The AI suggestions engine is continuously upgrading itself. As more customer interactions are logged, the model refines itself and improves its recommendations. This ensures that product recommendations are relevant, personalized, and fresh all the time.
Types of AI Suggestions (+Examples)
1. Search Recommendations
Search recommendations are product suggestions that are shown to customers as they type in their search queries in the onsite search bar. The AI recommendation engine generates these suggestions based on the search terms entered by the customer in real time. This includes products, product categories, articles, or simply search queries related to what the customer has typed so far. Besides search queries, this smart engine uses historical data, customer behavior, and popular product searches to suggest relevant results to customers.
Search recommendations are also referred to as search suggestions or autocomplete suggestions. It acts as a shopping helper for customers, automatically suggesting ideas even before they complete typing their query. By reducing the typing effort, it helps customers find what they are looking for faster, thus boosting the chances of making a sale.
Ambiance Boutique, a leading fashion store, uses search recommendations to enhance the shopping experience for its customers. As customers type into the store's search bar, the AI system immediately suggests relevant search queries as well as popular products. It often displays bestseller items offering customers a wide range of options, making it easier for them to make a purchase.
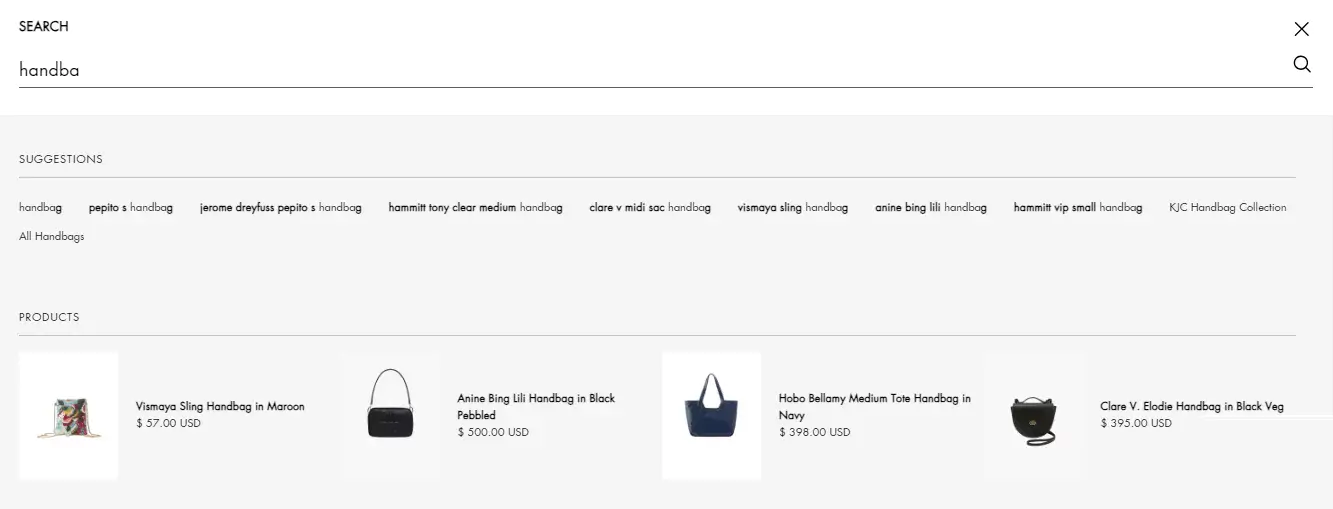
2. Personalized Product Recommendations
As the name suggests, personalized product recommendations are product suggestions that are tailored to individual customer's preferences, browsing history, and purchase patterns. It leverages the AI recommendation engine to understand which products would match the customers' interests and suggest only those products that they are most likely to purchase.
Instead of relying on generic suggestions, the AI suggestions engine focuses on what products are most relevant to each customer. Further, it is designed to dynamically refine its suggestions as per customers' current preferences. Thus, offering a knowledgeable sales assistant offering personalized service to every customer and enhancing customer experience.
Sephora, a leading beauty retailer, uses AI-powered personalized product recommendations to suggest makeup and skincare products to customers based on their past purchases and beauty profiles. Its beauty insider program contains interactive quizzes that capture important information about customers. For example, skin type, skin concerns, favorite makeup products, favorite brands, and so on. The AI suggestions engine uses this information while tracking customer interactions on the website to curate real-time, personalized recommendations.
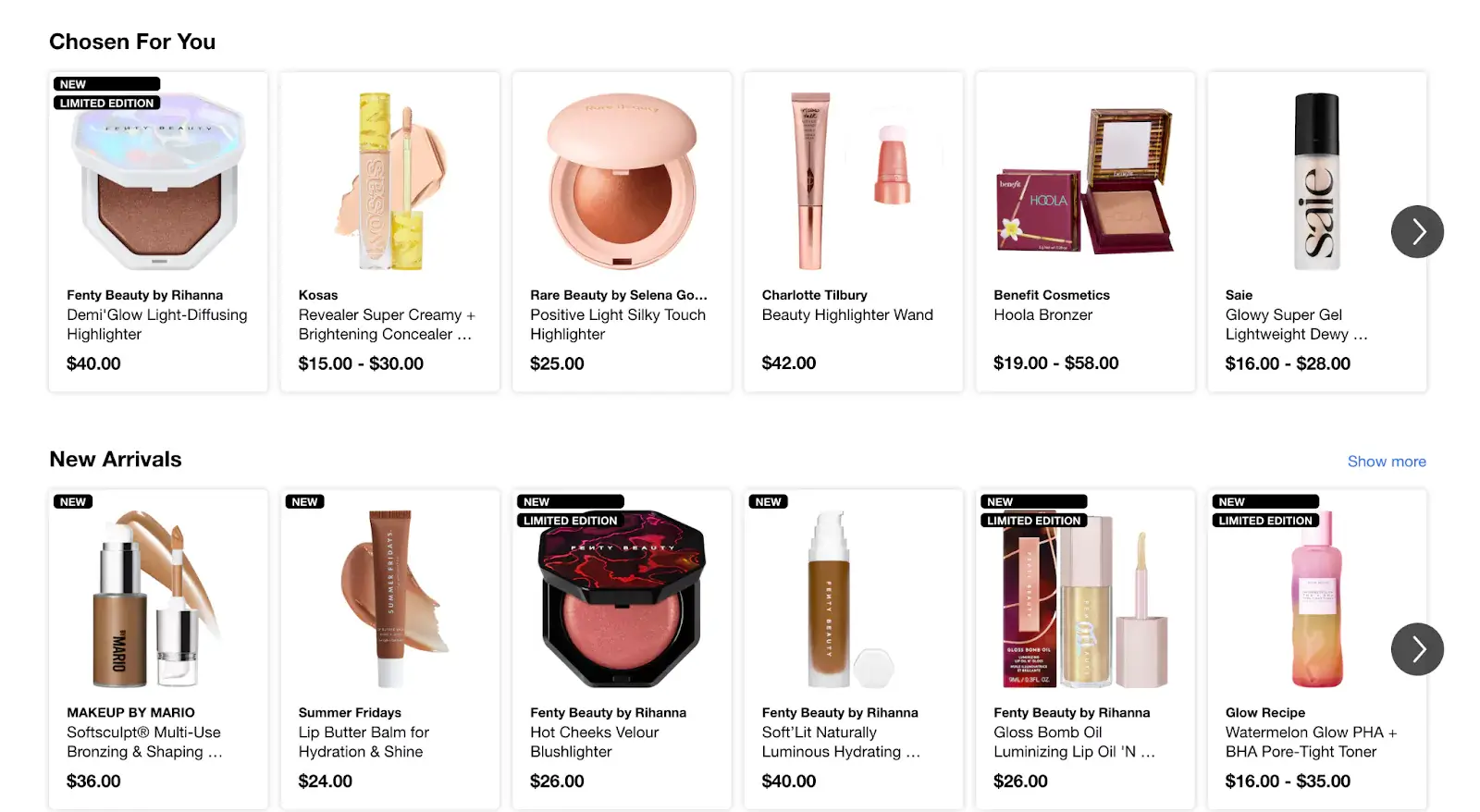
3. Upselling and Cross-selling Suggestions
Upselling and cross-selling suggestions are the best techniques to maximize the revenue generated per customer. And, leveraging AI to do so is like a cherry on the cake. Upselling with AI focuses on encouraging customers to purchase a higher-priced version of the product while cross-selling with AI involves recommending complementary products or related items based on the customer's purchase history or in-progress order.
The advanced AI recommendation engine analyzes vast data sets to identify customer preferences and patterns and tailor unique product suggestions that fit their needs or intended use cases.
Amazon leverages an AI suggestions engine to strategically implement upselling and cross-selling across the store. It identifies opportunities to present higher-end versions of products.
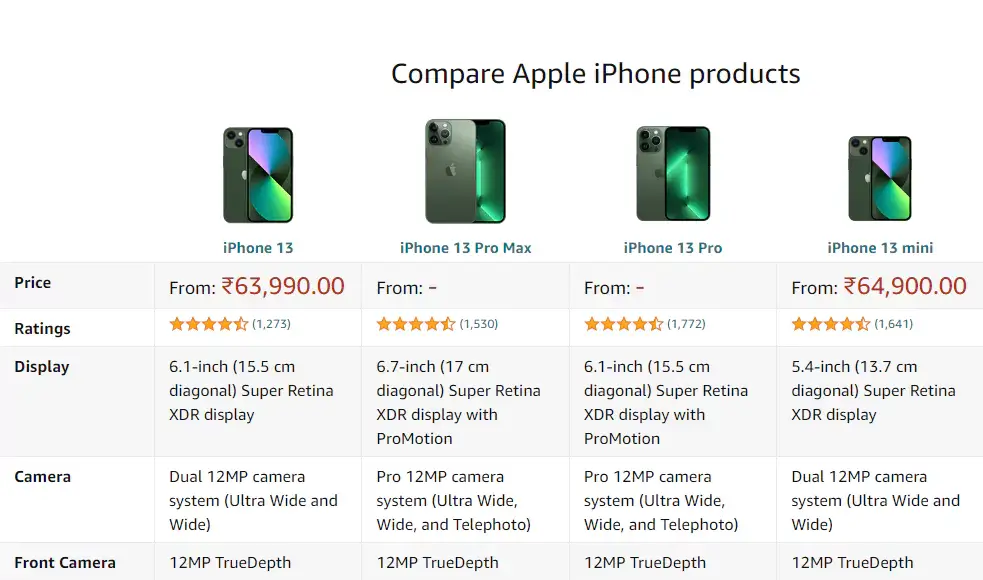
For cross-selling, it recommends complementary products that enhance the value of chosen products.
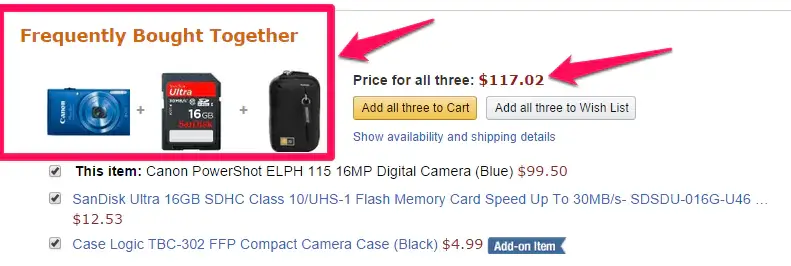
Thus, improving the shopping experience for customers while increasing revenue.
4. Related Product Suggestions
Related product suggestions are products similar to other products that customers are viewing. These product suggestions help customers to explore product alternatives or complementary products within the same category. The AI suggestions engine analyzes customer as well as product data to display contextually relevant items to customers, thereby boosting product discovery.
Samawa Perfumes, a leading perfume store, uses the AI suggestions engine to offer related product suggestions to its customers. These suggestions are not generalized recommendations but are generated by analyzing various product attributes and customer preferences. This approach has helped the brand to present a wide range of relevant products to customers, thereby reducing decision fatigue and increasing their cart value.
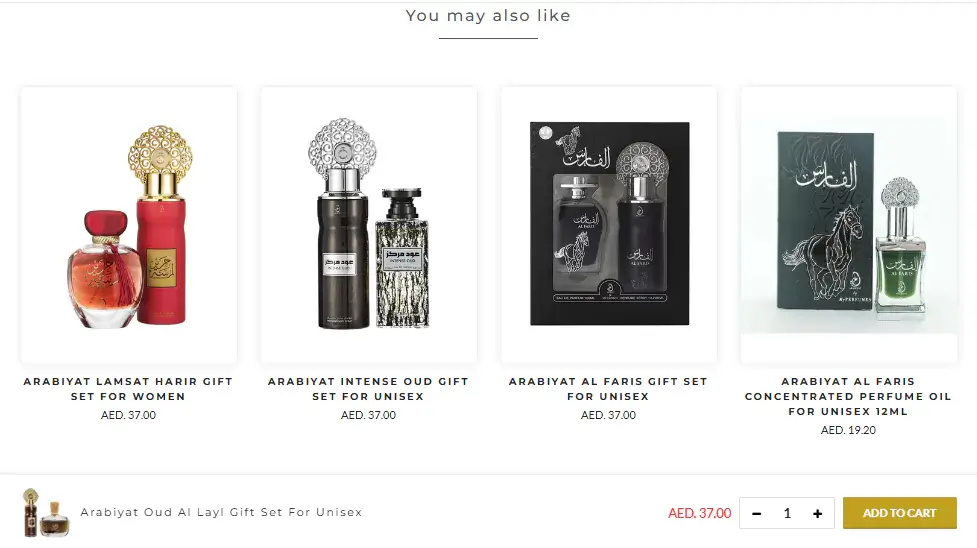
5. Customized Landing Pages
Customized landing pages are built by an AI suggestions engine to present hyper-personalized content to customers based on their behavior, preferences, and purchase intent. These pages are generated on the go and are optimized to highlight interesting products, ongoing offers, and engaging content that piques the customers' interests. This makes customers feel understood and valued, making seamless shopping experiences for driving faster purchase decisions.
ASOS, a fashion retailer, uses AI to build highly personalized landing pages for its customers. Based on the individual customer's preferences and behavior, it displays relevant products that align with customers' needs. As the entire webpage feels tailored, there is a spike in customer engagement that most often encourages quicker purchase decisions.
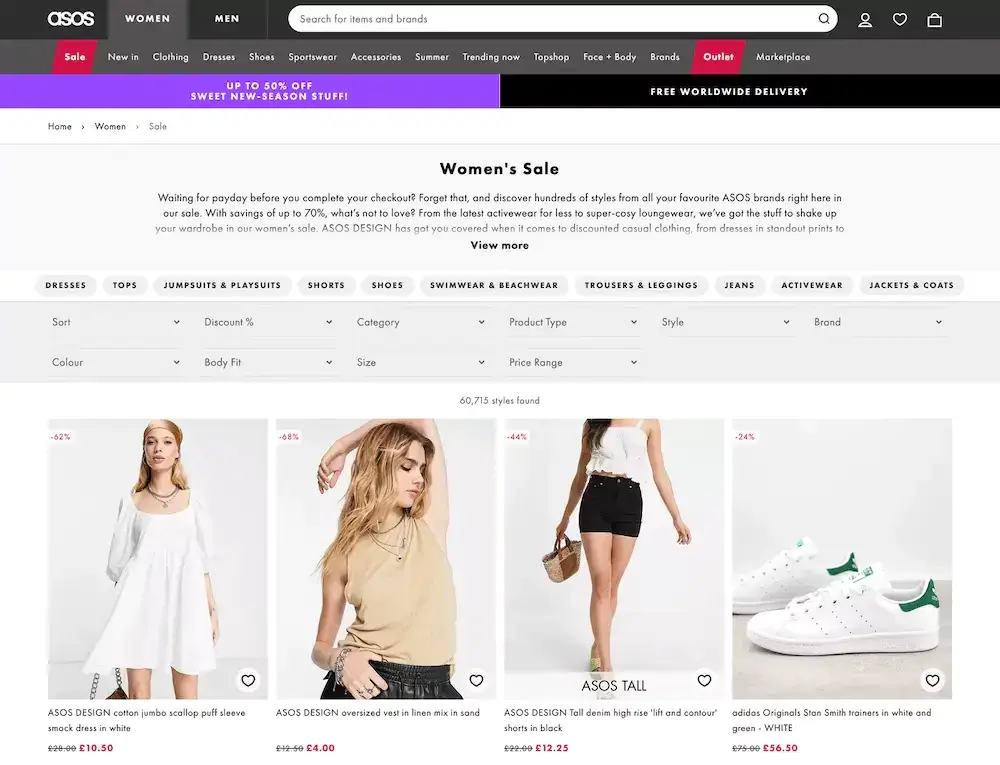
6. Checkout Suggestions
Checkout suggestions are product suggestions that are displayed to customers during the checkout process. These suggestions generally include complementary items, previously browsed products, items from the wishlist, or simply products that are relevant to the ones already in the cart.
Checkout suggestions are strategically designed to encourage customers to add additional items to their carts. It presents customers with last-minute opportunities to add more products to their carts without disrupting the purchasing process. Thus, offering a powerful tool for maximizing sales and improving customer satisfaction.
MyGlamm, a beauty retailer, uses checkout suggestions to increase order value. The cart or checkout page thoughtfully presents curated product options that complement the chosen item or bundle frequently purchased items together. This approach helps it maximize sales while making customers feel supported and valued during their purchase journey.
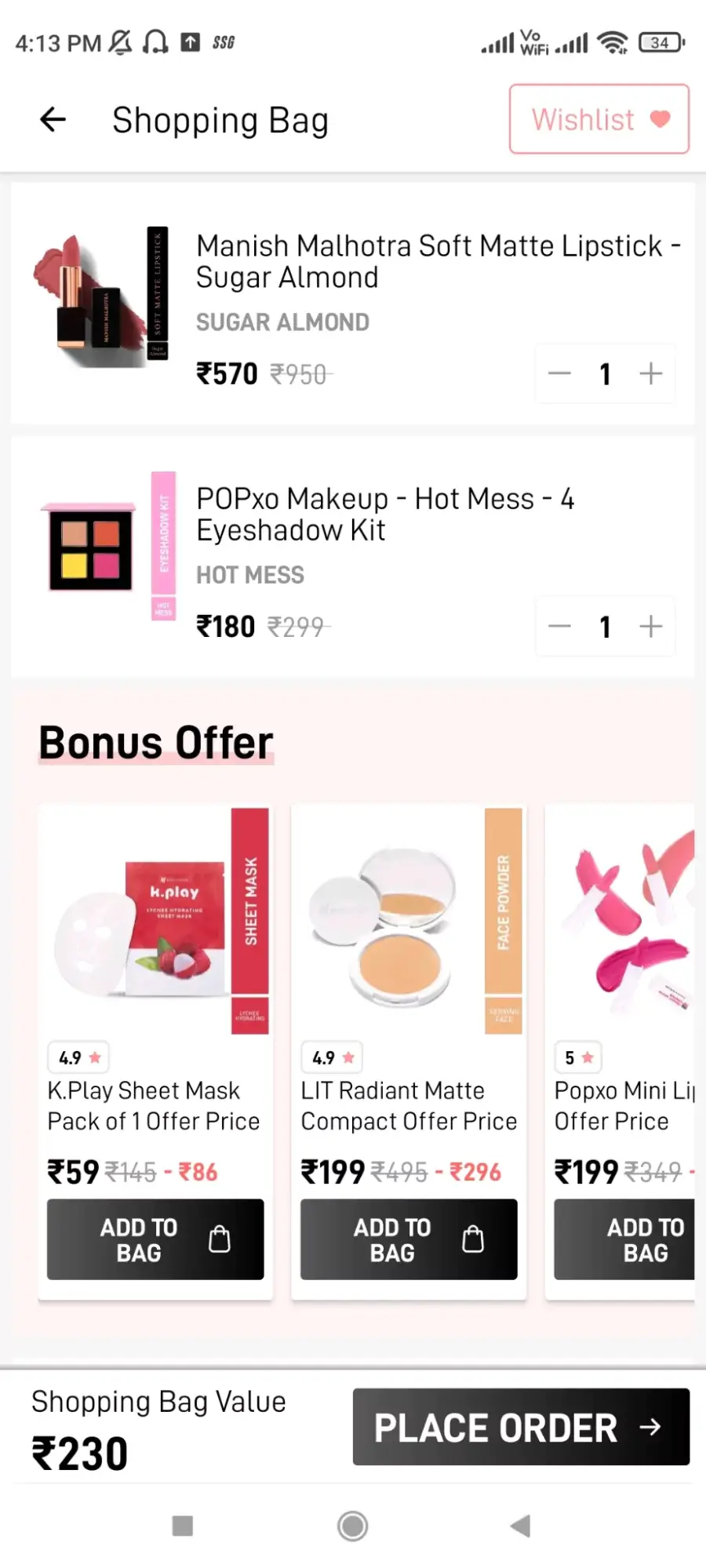
How to Implement AI Suggestions on Your Online Store?
Implementing AI suggestions in your online store can elevate the shopping experience, boost customer satisfaction, and increase revenue.
Sparq offers an incredible AI-powered product filter and search solution for online stores like yours looking to benefit from AI recommendations. It enables you to deliver tailored shopping experiences to customers with the help of its cutting-edge features like -
- Instant search as you type functionality
- Personalized product recommendations.
- Related product suggestions.
- Content suggestions.
- Predictive analytics for inventory management.
- Advanced reporting, analytics, and performance optimization.
With Sparq's AI-driven suggestions, you can create a personalized and seamless shopping experience for your customers. Sign up for a demo with Sparq and leverage AI suggestions to increase conversions in your eCommerce store.
FAQs
How does AI recommend products?
AI analyzes customer data like browsing history, past purchase history, behavior on your website, product preferences, and so on to suggest relevant products via highly personalized recommendations, each unique to every shopper's journey.
Do AI suggestions increase conversions in online stores? If so, how?
Yes, AI suggestions can definitely increase conversions. It can offer personalized product recommendations based on their interests, making it easier for them to find what they want. This encourages them to explore more items and complete their purchases, ultimately driving higher sales.
Can small businesses benefit from AI recommendations?
Yes, like large eCommerce retailers, small businesses can also benefit greatly from AI recommendations. They can leverage various AI tools to craft personalized shopping experiences to engage shoppers and convert them into loyal customers.
What are some best practices for implementing AI suggestions in my store?
To effectively implement AI suggestions in your online store, start by setting clear goals like boosting engagement or improving conversion rates. Integrate an AI-powered recommendation engine like Sparq to offer personalized product suggestions. Track and monitor analytics to understand what resonates with your customers the most.